During the past school year, we noticed a unique situation within one set of learners. But these diligent, eloquent, and hardworking online students were nothing else but bots. While these AI systems can produce poetry, summarize books, and respond to human-like queries, telling them 7 is less than infinity often leaves a lot of work unresolved. This article will delve into the reasons why this paradox exists and what efforts are now underway to rectify it.
The Language vs. Math Dichotomy AI chatbots like ChatGPT from the house of OpenAI are aimed at solving tasks related to language as per their design. They are used to generate text that makes sense, have a conversation with them as if you were talking to another human, or even write stories. On the contrary, their academic performance is mixed regarding mathematics. The good news is that these systems are trained to produce probabilities and make language from those sequences, not for exact formal deductions. In other words, something being probable does not necessarily make it true, and the fluidity of language juxtaposes against concrete mathematical statements.
AI’s Design and Limitations In short, “The AI chatbots suck at math,” quips Kristian Hammond, a computer science professor and AI researcher at Northwestern University who has not been involved with Socratic but read its technical white paper. In the past, computers were seen as “math on steroids”. Ideal for numerical problems that humans could never work through. These were methods in which an early desktop film will adhere to rigid step-by-step laws as well as draw facts from a nicely-authored database. They were strong but easily broken—a common brick wall in developing AI.
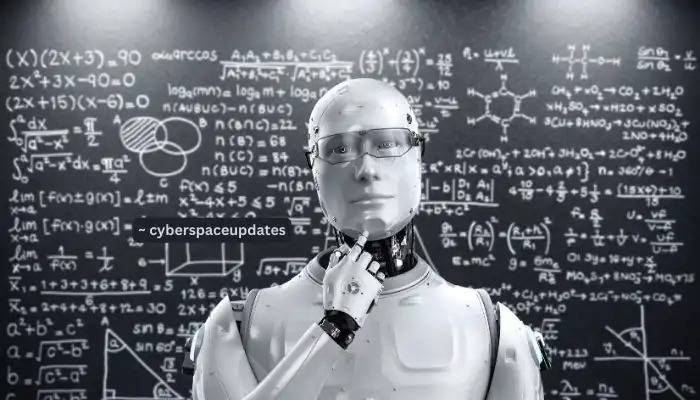
Over a decade ago, the advent of neural networks (roughly modeled after how human brains operate) brought about the modern approach to AI. Instead, the technology can be taught through a vast body of data rather than inflexible programming. It produces by predictive speech means, i.e., it predicts the most probable word/phrase to follow up based on whatever information fed into it, like how a baby learns words. This has its merits no doubt, but at the same time, there are limitations.
Math AI Growing Pains The common joke—math problems confound an innocent chatbot. But as Kristen DiCerbo, Chief Learning Officer of Khan Academy, pointed out at a recent symposium, this is the conundrum that we are facing with math accuracy in artificial intelligence systems. In turn, Khan Academy itself reconfigured its AI tutor, known as Khanmigo, moving a lot of the numerical work onto an off-screen calculator program—presumably labeling that “doing math” whilst it crunched away. Likewise, ChatGPT has been using a hack for many math questions for over a year based on the calculator program; it can use a calculator to divide and multiply large numbers. OpenAI points out that mathematics is still a “major area of ongoing research,” and the most recent GPT model has nearly reached 64 percent accuracy on the set compared to only around 58 percent for earlier versions.
The Debate on AI’s Future Path The AI community is debating the best course of action in designing and deploying future AIs. One school asserts that it simply requires an advanced neural network, the large language model (AGI), to achieve artificial general intelligence; a computer with all human brain functions. This world view is overrepresented in Silicon Valley. In a symmetrical view, skeptics like Meta Chief AI Scientist Yann LeCun claim that big language models are devoid of logic and common-sense reasoning. LeCun promotes “world modeling,” systems that can build an understanding of the world in a manner similar to humans, estimating it might take another decade to do this.
Practical Applications and Real-World Impact Despite their flaws, current AI models are widely used. David Ferrucci, the computer scientist at IBM who led that company’s group in creating Watson, said computers have come a long way toward understanding natural language with all its ambiguity and variations, but they still fall short of being as precise as needed for applications like banking or air traffic control. At his latest startup, Elemental Cognition, he marries large language models with more rules-based software to improve decision-making in areas like finance and drug discovery.
Kirk Schneider, a high school math teacher in New York, thinks the integration of AI chatbots into education is inevitable. While administrators may try to “ban them,” students will use drones. Schneider introduces chatbot feedback as a pedagogical device, urging students to deeply analyze their own answers against the AI’s and maintain critical thinking with respect to its advice.
Conclusion: A Lesson for Life The struggles of AI chatbots with math serve as a great example of why we need human guidance and a reasonableness approach. When students learn to ask and check AI-generated answers, they are learning skills that are also useful beyond the classroom. The moral of the story: do not rely too much on AI and always verify its outputs. Artificial Intelligence needs a human touch: In the future, AI in education and all other fields will depend on how tentative they are when it comes to keeping the perfect balance between technology enhancement and the precision of humans.