AI and ML technologies generally as well as Generative AI in particular, are predicted to have an enormous impact on different sectors within India during the next 10 years. In accordance with the EY study, Generative AI could boost the GDP of India by 359-438 billion by 2030. This is an rise of 5.9-7.2 percentage increase which could bring an overall US$1.2-1.5 trillion to the economy of India through the FY 2029-30. Based on the MeitY report IndiaAI, AI is expected to add $500 billion to India’s GDP in 2025. making up 10% of the nation’s goal of a GDP of $5 trillion which is expected to increase the value of $967 billion in the Indian economy before 2035.
The improvement in employee productivity, efficiency in operations, and a high level of customers’ engagement are major factors in this effect. Yet, about 75% of businesses have the lowest to middle level of preparedness to reap the advantages that come from Generative AI. How can companies benefit from AI to boost their performance and remain ahead of the curve? The process of implementing AI isn’t an easy job. It involves meticulous planning, execution and evaluation in order to assure that AI will deliver the payoff you want and adds value. Let’s take a look.
1. Definition of the Business Goals
The initial step in integrating AI in your company is to identify your company’s goals and determine the areas where AI could be beneficial. Generative AI technology, for example are able to benefit firms “generate” words images, speech and videos, as well as music and even code. It will significantly benefit businesses by automation of material production, optimizing supply chains, and improving the quality of customer service.
The most important question, then what is the goal you want to achieve in AI application, since AI can be used to serve a broad array of tasks. There is a need for an accurate idea of your goals for business as well as the challenges and opportunities as well as map them out to possible use cases and the benefits of AI. This will benefit to prioritize your AI projects in line with your overall business plan, and assess their effect.
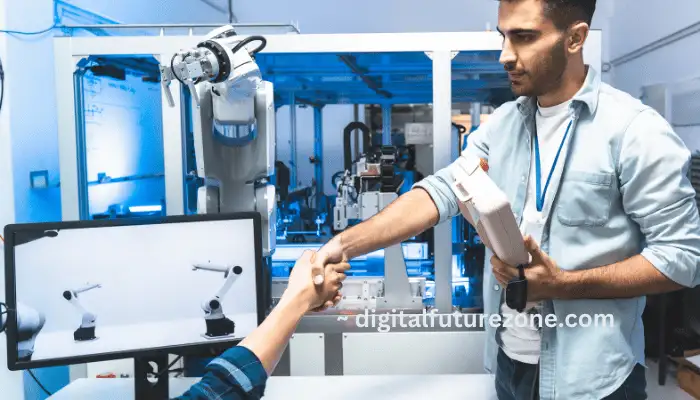
2. Choose the Correct Data Sources
The next process is to determine relevant data sources within your company and assure they’re reliable and up-to-date. and easy to work with. Data is vital to AI since it provides the base for training AI models, which allows them to increase, change to, and then make choices based on information they’ve received. The synthetic data may benefit in the event that data is lacking or is scarce. It could be utilized to augment information used to train models in order in order to boost the reliability and accuracy in AI models.
The most important aspect to your AI strategy must be an approach to data collection from reliable sources, data processing and integration in a secure and efficient data storage systems, as well as the ability to identify and resolve concerns about the quality of data.
3. Pick the right AI stack
The next step is to select the best AI stack. This comprises the set of frameworks, tools, and models that you employ to create, deploy and maintain the deployment of your AI solutions. Ideally, this requires the use of open-source AI models, techniques that are modern AI pipelines that are able to handle the demands of. Relying on closed models or proprietary software reduces the flexibility of your system as well as causes long-term lock-ins and is a major risk to your business. In contrast open source models are at your disposal and permit you to build your platform in a manner that is in line with your needs.
Different versions of LLMs like Mistral Llama2 and Falcon LLMs are a great way to address issues related to material generation, develop chatbots to assist customers or comprehend your client’s data or documents. When it comes to image generation, you should consider options such as Stable Diffusion; for video generation, consider Stable Video Diffusion; and to synthesize audio, use Meta’s AudioCraft. Open-source speech recognition and voice synthesis software are also extremely effective. There is also the possibility of requiring an Vector Database or Knowledge Graph to build an AI pipeline which can make use of information from your organization as well as deliver some context for an AI model.
4. Pick the right infrastructure
The final step in integrating AI to your organization is choosing the appropriate infrastructure to manage your AI applications. Choose the most appropriate AI-centric Hyperscaler could have a major influence on the performance and return on investment of the AI pipeline as it will give you advantages over common-purpose Hyperscalers. In particular that the Hyperscaler will be able to give you extremely efficient cloud GPUs, as well as cloud GPUs, such as the high-end HGX H100 that is backed by InfiniBand as well as sophisticated cloud GPUs, such as the A100 and L4OS and others.
The right AI platform can be the difference between success and failure for your AI plan, because it can have a direct effect on costs and could make the AI platform highly effective. Also, you should think about the region that the Hyperscaler operates from. As AI frequently handles sensitive corporate data It is essential to assure that the data you store on the cloud is secure from intruders by foreign entities and in compliance to the laws and regulations applicable to Indian geographical areas.
5. Design, train and then test
Fifth step: build, train, and evaluate the AI solution, which is the process of developing the, refining and then checking and validating your AI models. The process entails analyzing aspects of data, breaking it down into testing and training datasets before adjusting the AI models or constructing AI pipelines that integrate the data from your business to AI’s database of knowledge. This is essential because it will benefit to customize your AI system alike to the business goals you have and benefit create outcome which work in real-life situations. Additionally, you must evaluate your AI models and adjust your approach based on outcome.
6. MLOps and Production Deployment
The final step for integrating AI in your company is to put your AI products in production that is creating your AI models accessible to customers or end-users. This involves packing and deploying your model to be used for inference and serving users of the model through the integration of the application stack or APIs.
For you to implement your AI applications on a regular basis effectively and efficiently, it’s important follow a set of desirable methods and guidelines, also known as MLOps which refers to an application to apply DevOps for machine-learning. MLOps is designed to simplify and automate all aspects of the AI cycle, from creation through deployment, maintenance and upkeep as well as facilitate collaboration and coordination across different roles including engineers, data scientists, as well as business analysts.
7. Monitor, refine and Update
The next stage is to track your, modify, and improve the quality of your AI solutions. This means maintaining your AI products relevant, productive and in line with your goals for business and user demands. The process involves studying the behavior of users, training or altering AI models with the latest information. This is to continuously adjust and increase the AI models to ensure that they boost with time in terms of accuracy.
Last Words
While the AI market grows and businesses who are proactive about and improve their journey to transformation are likely to recieve an edge in the market by staying on top of technology and harnessing the full power of AI technology. It is important to plan prior to time as we enter the AI decade where significant shifts in business models and workflows are anticipated. In doing this, they will can remain competitive while reducing risk for the future.